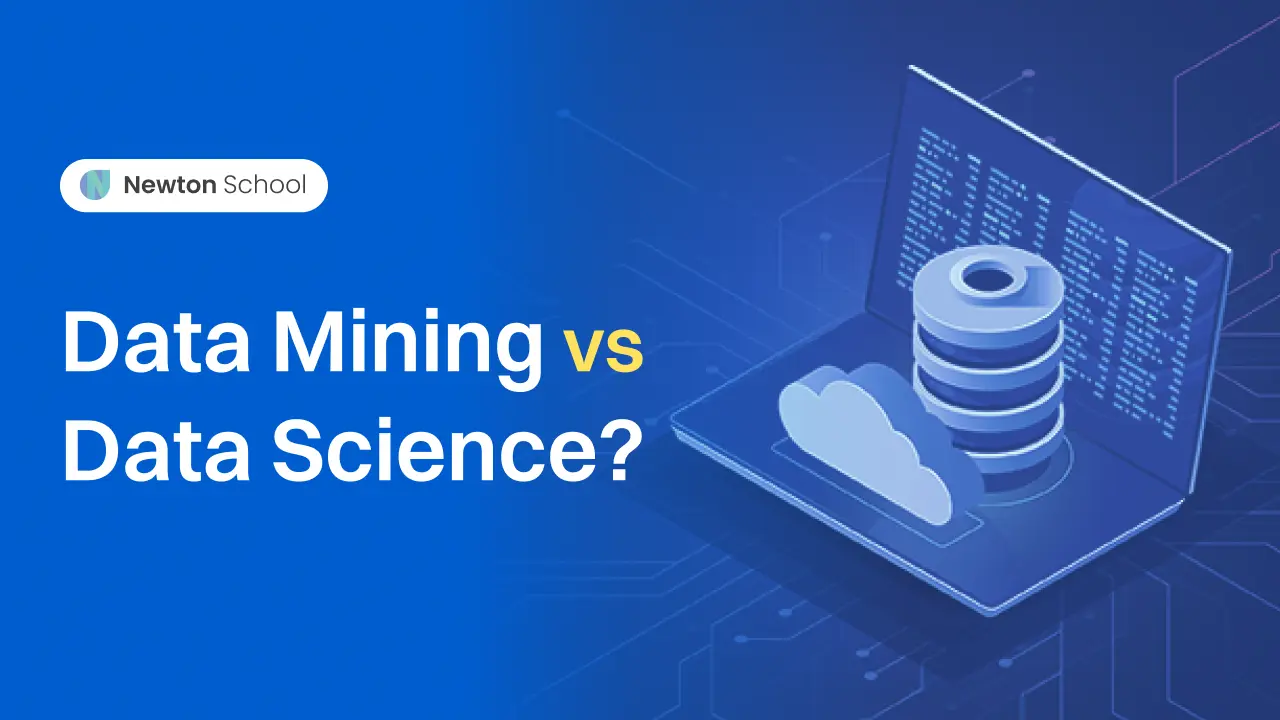
Storing texts, reports, and substantial information is not unique to the 21st century. For centuries, libraries, historians, and archivists have stored data in fine print. However, the process, purpose and volume of data have changed dramatically. Every month, seven billion users log on to the Internet for various activities. Every move we make on the Internet is tracked. Every site we log on to and every image we engage with is stored in the form of caches and cookies. All of this is referred to as data.
Before we dive deep into the glaring differences between data mining and data science, we must clarify that there are no textbook definitions of both, as they are developing fields.
Definition
When data is presented in huge numbers, it becomes impossible to comb through significant portions and turning points of the pile to determine a cohesive trend.
Data mining
Data mining is the process of sorting through extremely large data sets to identify repetitions and patterns that can assist in solving a business problem. The process also helps in predicting future trends and making reliable decisions that save time, money, and resources for a business.
Data science
Data science is a broad field that covers the tracking, extraction, extrapolation, and structure of data.
Terminology and usage
Data science, the broader science of data management, is an umbrella term that covers a multitude of processes that deal with data. It can be best described as data-driven science that deals with obtaining and analysing data and extracting information from it.
Data mining, on the other hand, is a sub-field of data science, which is all about discovering data and making cohesive patterns out of a maze.
Although both appear to be contemporary, data science has been around since the '60s, while data mining is a rather novel term, which started gaining steam during the dot-com bubble of the '90s.
Key differences between data science and data mining
Data mining and data science are leading fields of resource allocation and are an essential part of today's data management. There are significant differences in the way they are discussed, approached, and applied in real life.
Here's how:
Data mining vs data science: Purpose and application
Data mining and data science: Career and job scope
Data mining is a job for people who can navigate data and understand the process of analysing large blocks of data and extracting meaningful trends. It is a specialist position performed by data mining engineers. However, data scientists are full-stack engineers and experts, also known as data scientists, who are experts in data programming and data management.
Data mining vs data science: Learning and education
When it comes to choosing a study path, both data mining and data science are worthy of consideration. Once you have the proper understanding of both, it will be easier to make a decision.
Now you have a clear idea that:
- Data science is a chosen field of study, whereas data mining is one of the streams of the field.
- Data mining is part of the broader process that constitutes data science.
- While data science is a wide field of study, data mining is a specialization.
- Data mining is a subset of data science.
- Other processes included in data science are pattern recognition, statistical analysis, and data flow.
So, if you are inclined toward using data sets to identify patterns and discover hidden figures, then data mining is the perfect field of study for you. However, if you like to work with structured and unstructured data sets, then you should pursue data science.
Data science is an entirely different field of study, of which data mining is a part.
Every single day, we add 2.5 quintillion bytes of data to the internet. It is an unfathomable amount, and yet, businesses around the world have figured out how to use it to their benefit, thanks to data scientists and data mining engineers. Data science and data mining are two of the most essential concepts of modern data management and cannot be used interchangeably.